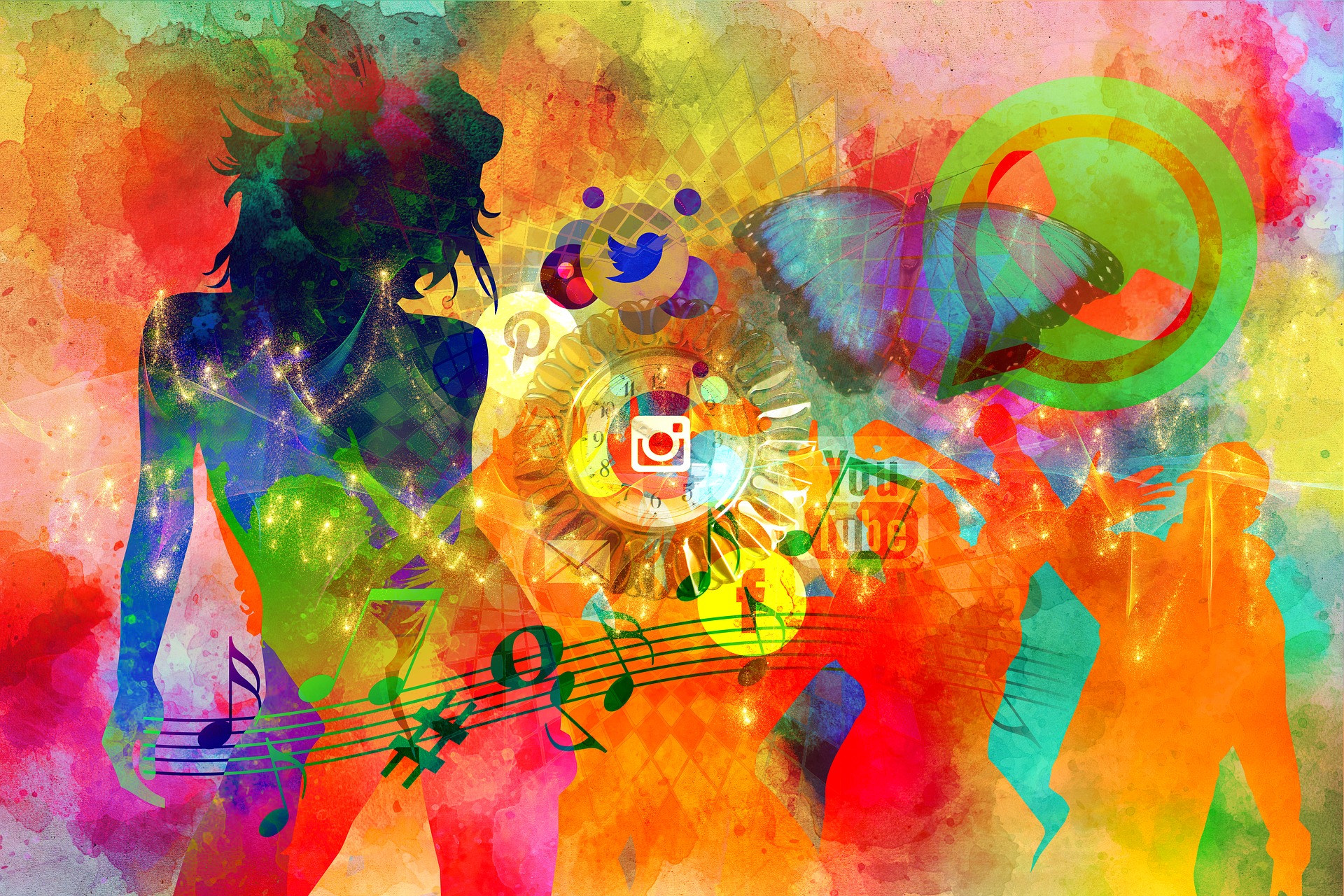
Introduction
This two-part blog post offers a reflection on the topic of content moderation and bias mitigation measures in copyright law. It explores the possible links between conditional data access regimes and content moderation performed through data-intensive technologies such as fingerprinting and, within the realm of artificial intelligence (AI), machine learning (ML) algorithms. More specifically, this post explores whether current EU copyright rules may have the effect of favoring the propagation of bias present in input data to the algorithmic tools employed for content moderation and what kind of measures could be adopted to mitigate this effect.
Our analysis explores the dynamic of “bias propagation” in relation to the obligations stemming from Article 17 CDSM Directive. In simple terms, Article 17 incentivizes certain platforms to filter content uploaded by users to comply with their “best efforts” obligations to deploy preventive measures against infringing content. Prior to the introduction of this legal regime, however, some platforms already “voluntarily” relied on similar automated content moderation (e.g., YouTube’s’ ContentID). At the current state of technology, filtering appears to be done mainly through matching and fingerprinting. However, these tools are incapable of assessing contextual uses (see, e.g. here). Therefore, they are not suitable to ensure the required protection of freedom of expression-based exceptions like parody, criticism and review, as required by Article 17(7). Accordingly, more sophisticated tools seem necessary to enable preventive measures while respecting users’ rights and freedoms, as recently confirmed by the CJEU in case C-401/19 (see here). This suggests that ML algorithms may increasingly be employed for copyright content moderation given their alleged superiority in identifying (understanding?) contextual uses.
Against this background, a crucial question emerges for the future of online content moderation and fundamental rights in the EU: what happens when these tools are based on “biased” datasets? More specifically, if it is plausible that any bias, errors or inaccuracies present in the original datasets be carried over in some form onto the filtering tools developed on those data: (1) How do property rights in data influence this “bias carry-over effect”? and (2) what measure (transparency, verifiability, replicability, etc.) can and should be adopted to mitigate this undesirable effect in copyright content moderation in order to ensure an effective protection of fundamental rights?
Part I of this post briefly discusses the concept of bias and examines the role of property rights in data and factual information, with a focus on copyright. Part II explores the potential of property rights to increase bias in content moderation by looking at the topic from the perspective of Article 17 CDSM Directive.
Bias, data, and property rights
Looking at the common meaning of the word, bias may be defined as the tendency to favor or dislike a person or thing, especially as a result of a preconceived and often unfairly formed opinion that affects behavior. In scientific literature, bias is usually defined in relation to a specific field, area or group (e.g., cognitive bias, algorithmic bias, confirmation bias, gender bias, etc.). Common denominators are the presence of an error that is due to systematic imprecisions, or to deviations from standards in judgement which lead to unfair, inaccurate, illogical or discriminatory conclusions. Wikipedia lists more than 200 types of bias, just within the field of cognitive sciences.
In the field of AI, bias may be present at various stages of the development of an application including the design of the algorithms, the designers of the algorithm, the identification and sampling of the learning information and the curation, annotation, and verification of the input data (see, e.g., here and here). Bias in AI may be particularly treacherous due to the specific technical and societal characteristics that this technology has acquired. On the one hand, it is often said that AI operates as a black box in the sense that it is not possible for humans to really understand the learning process that happens within the algorithm and therefore to detect bias and inaccuracy by employing traditional approaches. On the other hand, given the widespread adoption of AI in our society and the fact that in an increasing number of cases these applications rely on the same or similar pre-trained models (i.e. already processed input data, such as BERT, GPT-2, GPT-3 in the Natural Language Processing (NLP) sector), any bias present in these pre-processed datasets may be transferred to all implementations of that system giving rise not only to some sort of “bias carryover” but also “bias multiplication” effect.
The focus of our analysis is on those elements of the EU copyright acquis that create conditions for access and reuse of non-personal data and that could therefore play a role in the propagation of bias. In doing so, it is important to highlight some key traits of the EU acquis. First, when we refer to input or training data (a technical category) we mean material that from a copyright law perspective could be either works of authorship, other protected subject matter or mere facts and data. Second, although mere facts and data as such are not protected by copyright, their extraction from protected subject matter (works, non-original databases, etc.) often requires authorization under EU copyright law. Third, exceptions and limitations for the extraction of information from works are narrower in the EU than in many other non-EU legal systems (see e.g., here). Fourth, as a consequence of the above, EU copyright law can be said to protect information needed as input data for AI applications to a greater extent than other legal systems (see e.g., here).
The mechanism by which these key traits of EU copyright law may influence bias is relatively simple. By regulating access to training data through the imposition of costs or other use conditions, property rights may create unanticipated incentives that drive AI developers towards “more available”, “cheaper”, “less risky” or as it has been called “low friction” data (Levendowski 2018), which incidentally are more easily found outside the EU. However, whether these data represent the optimal choice in terms of quality, accuracy, and representativeness or whether they are simply chosen to reduce the economic, informational, or legal certainty costs, regardless of their suitability for the task, is far less clear.
Attempting a first categorisation of “data types”, the following five scenarios may be logically derived from an application of the EU acquis to the specific field of input or training data.
Scenario (1): Public Domain
The public domain is arguably the “cheapest” source of data. The main problem with this category is that to enter the public domain underlying works are on average at least 70 years old, and often much older. Accordingly, there is a risk that data extracted from this source may likewise convey outdated, disproved or surpassed information.
Scenario (2): Open Licenses
Open Licenses are likely the closest scenario to the Public Domain in terms of “costs”. Tools such as Creative Commons licenses (e.g., Wikipedia), GNU General Public License (e.g., Free and Open Source Software) and open government licenses (e.g., reuse of Commission documents) play a major role here. However, openly licensed information is not necessarily immune from bias just for being openly available, even though openness certainly allows for greater transparency and thus closer scrutiny of the underlying dataset.
Scenario (3): Exceptions and Limitations
Next in terms of “costs” is information accessible thanks to exceptions and limitations (E&Ls) allowing data analytics or computational uses (e.g., text and data mining or “TDM”). As mentioned above, under EU law, exceptions for TDM purposes or use (in Articles 3 and 4 CDSM Directive) are arguably narrower than in other jurisdictions, such as the US, Canada or Japan. Accordingly, the training of AI systems in the EU is conceivably more “expensive” which, in turn, operates as an incentive for the training of AI systems in “cheaper” jurisdictions or to import from those jurisdictions “pre-trained” models. The deeper implications of these dynamics are far from clear. For instance, will a ML algorithm trained in the US learn the meaning of a given concept, e.g., “parody”, within the US socio-cultural context and then apply that meaning in the EU when asked to perform a function such as “filter copyright infringing videos”? Is this technically plausible? If yes, what impact could this have in an online regulatory environment that is increasingly relying on privatized algorithmic enforcement?
Scenario (4): Third party content
When the above scenarios are not applicable or deemed not adequate by virtue for instance of a cost-benefit analysis, access to the information needed for training purposes may follow “traditional” contractual arrangements. Two main situations may be envisaged. Firstly, the required training information may already be hosted by the entity interested in the training, as it is arguably the case for all major Internet platforms hosting large amounts of third-party content which is licensed in a way that usually allows the platform to develop its own services. Secondly, the entity interested in the training needs to acquire access to third party content often hosted in large commercial databases, examples of which may be scientific commercial databases offering TDM licenses to commercial or academic users. This scenario seems to favor the strengthening of the dominant position of large platforms which will not need to pay the extra price to train on third party content they already host, a price that conversely other, usually smaller, players who do not own these large databases will need to pay thereby further reducing their competitiveness in this market.
A third case may be conceived where right holders are compelled to contribute “relevant and necessary information” about their content to qualifying platforms to develop dedicated filtering tools, as provided in Article 17(4) CDSM Directive. This is an interesting development, which however requires a dedicated analysis. Among among other aspect, such analysis will need to distinguish among the technologies adopted i.e., fingerprinting and hashing, or ML algorithms, and assess the specific role of the provided data.
Scenario (5): A risk-benefit analysis leading to opacity
Finally, it is at least plausible that EU-based firms developing AI systems decide to perform training activities regardless of all the above considerations, knowing that it is unlikely they will be “discovered” engaging in potentially copyright-infringing activity. Once trained, especially employing modern algorithms that reach deeper levels of abstraction, it will be difficult to “reverse engineer” the models, i.e., to go back from the model to the training data and therefore to demonstrate infringement. This scenario – the plausibility of which should be further tested – may lead to an increased opacity in the training process: firms will have strong incentives not to disclose details about the training sources to avoid acknowledging their own infringement of third-party rights.
In conclusion, the identified “costs” associated with the uneasy case of property rights in data have the potential to favor the use of outdated and lower quality data sources, or to disincentivize transparency and accountability in the training process, all ideal conditions for bias and errors. This will push AI developers in high-cost data legal systems, such as the EU, to either outsource data analytics to non-EU legal systems – with the unexplored consequences on fundamental rights and cultural dynamic sketched above – or to be even more opaque in their data policies with similar negative consequences on fundamental rights and freedoms.
Following the above discussion of the concept of bias and the role of property rights in data and factual information, with a focus on copyright, the second part of this post will explore the potential for property rights to increase bias in content moderation. We will do so by looking at the topic from the perspective of Article 17 CDSM Directive.
Acknowledgments: This research is part of the following projects. All authors: the reCreating Europe project, which has received funding from the European Union’s Horizon 2020 research and innovation programme under grant agreement No. 870626. João Pedro Quintais: VENI Project “Responsible Algorithms: How to Safeguard Freedom of Expression Online” funded by the Dutch Research Council (grant number: VI.Veni.201R.036).
This blog post is based on the EPIP2021 roundtable organised in Madrid (September 8-10, 2021). The authors are grateful to Prof. Niva Elkin-Koren and to Dr. Irene Roche-Laguna for their participation and for their insightful perspectives and suggestions which have been helpful in developing this analysis. The blog post only reflects the view of the authors and any errors remain our own.
________________________
To make sure you do not miss out on regular updates from the Kluwer Copyright Blog, please subscribe here.
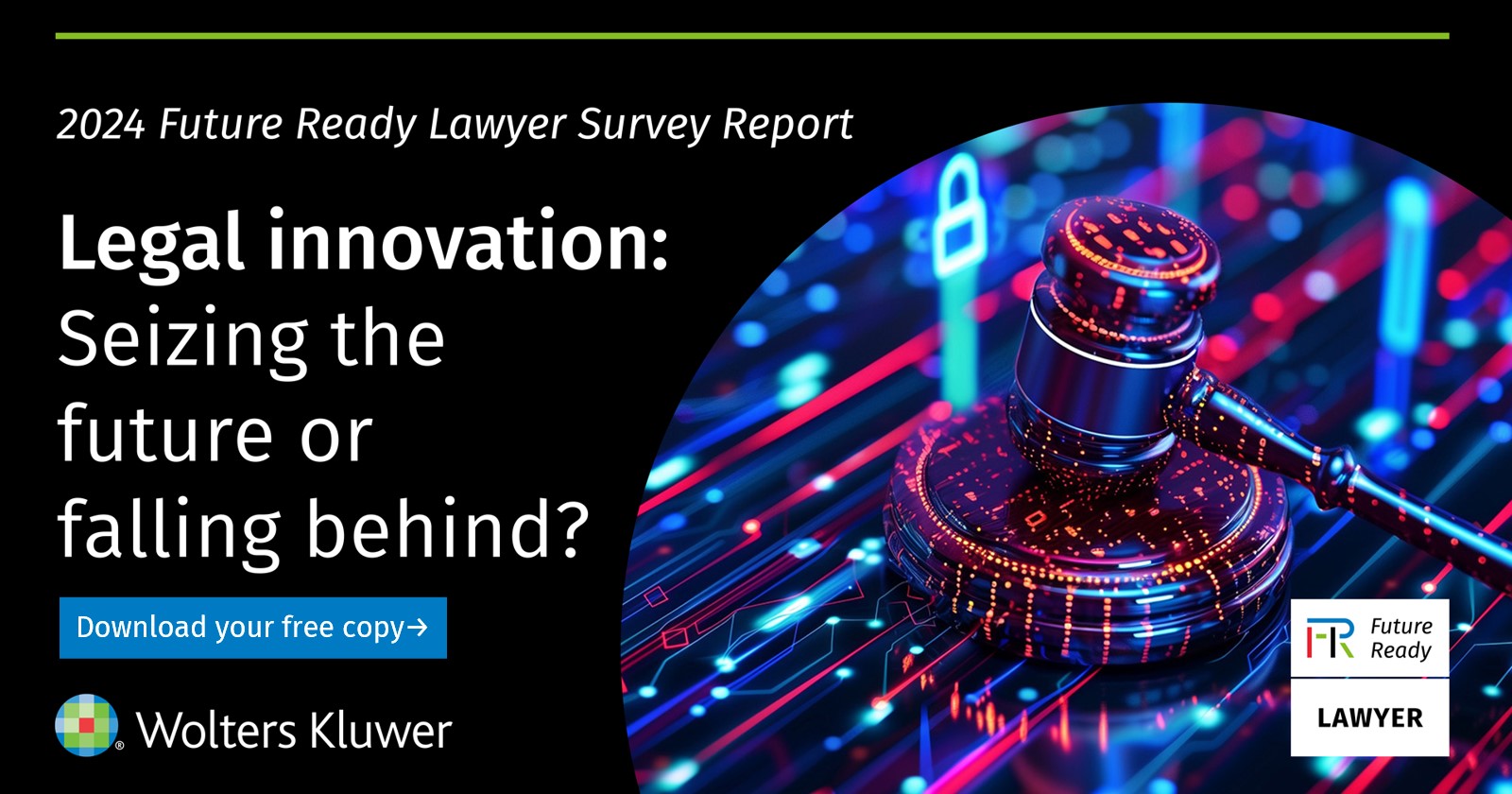
