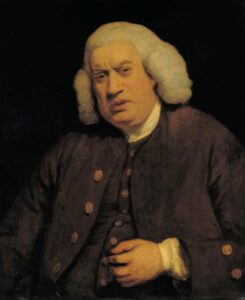
Large language models are built on scale. The bigger they are, the better they perform. The appetite for letters of these omnivorous readers is insatiable, so their literary diet must grow steadily if AI is to live up to its promise. If Samuel Johnson, in one of his famous Ramblers of 1751, grumbled about the growing number of what he called “the drudges of the pen, the manufacturers of literature, who have set up for authors”, who knows what he would be saying about these large language drudges? We can speculate as much as we like, but one thing is certain: they, too, are hungry not just for any data, but especially for well-crafted data, high-quality texts. It is little wonder that Common Crawl, a digital archive containing some 50bn web pages, and Books3, a digital library of thousands of books, have become widely used in AI research. The problem is that high-quality texts have always been in short supply, not just in the age of Johnson, and this is a bottleneck that has sparked a lot of concern in the AI industry. Epoch AI, a research firm, estimates that the total effective stock of human-generated textual data is in the order of 300 trillion tokens, and if AI models with 5e28 floating-point operations continue to be trained “compute-optimally”, the available data stock will be exhausted in 2028. This is known in the industry as “data wall” (see here and here).
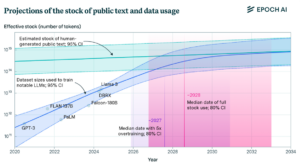
But what will happen when we hit the data wall? Well, there is no definitive answer to that and, again, speculation abounds. One possibility is that, as we race towards the wall, high-quality texts will become ever more valuable to starving LLMs. This could give a whole new momentum to the copyright policy proposal to refine the opt-out vocabulary of possible uses of copyrighted material, thus providing authors with opt-out options that are more granular, e.g. providing licencing options, than the binary approach of either opting out of all text-and-data mining (TDM) or declaring no opt-out at all (see here).
Another avenue to explore could be the use of synthetic data as grist for the AI mill, i.e. using curated and high-quality synthetic data as training material. This alternative has some advantages. Real data is hard to come by and expensive to label; using synthetic data instead is not only cheaper but also promises to sidestep the thorny issues of privacy and copyright infringement (see Lee 2024).
But there are also important drawbacks to consider. The biggest concern with synthetic data is quality. Producing high-quality synthetic data is not as easy as it sounds on paper. In information theory there is a principle called “data processing inequality”, which roughly means that any data processing can only reduce the amount of information available, not add to it. Put simply, as new synthetic data comes in, a better version of it comes out (see Savage 2023). Even synthetic data that comes with privacy guarantees is necessarily a distorted version of the real data. So any modelling or inference performed on these artificial sets carries inherent risks (see Jordon et al. 2022). Another potential problem is that once synthetic data becomes the only game in town, its owners will be keen to claim copyright on it – or other forms of property – and litigate against other players in the AI business to protect their product. This would undermine the most promising aspect of synthetic data: its potential to redress the market imbalances in data access and democratise AI research. Moreover, the use of synthetic data in training sets may avoid copyright infringement, but only so long as the production of the synthetic data itself has not infringed any copyright. If it has, then training machine learning systems on that infringing data “may not resolve issues of copyright infringement so much as shift them earlier in the supply chain” (see Lee 2024). After all, every piece of synthetic data has a human fingerprint at its core.
Which leads us to a final point of a rather philosophical nature: reliance on synthetic data will only hasten the transition to a post-human order of creativity, potentially shattering the core notions of originality and authorship that we have cherished since Romanticism and that remain deeply inscribed in the Promethean DNA of modernity. At the risk of betraying an unintentional cultural pessimism, it might be worth considering whether the human prerogative of supreme creativity is something we would be willing to negotiate or sacrifice on the altar of technological progress. If LLMs are stochastic parrots (see Bender, Gebru et al., 2021), wouldn’t relying on synthetic data amount to an eternal repetition of all things created by humans? Wouldn’t we just be replicating the past or, as I once heard a neuroscientist say, producing a “future full of past and barren of future”?
But as the pool of publicly available data dries up, copyright is likely to face yet another difficulty in the coming years that we might call the “quantum challenge” (see here). The cybersecurity architecture of our data communications – essentially what underpins the global economy – is based on encryption systems that rely on the factorisation of huge numbers. The mathematical principle behind this is not a hard nut to crack: multiplying numbers can be easy, but factorising large numbers can be prohibitively difficult. This is an example of a “one-way function”. If you have ever made an online payment or sent a WhatsApp message, a one-way function has been used to secure your data. This is where quantum technology comes in: while it would probably take a good many years to factorise a 600-digit number using classical computers, an enhanced quantum computer equipped with an algorithm like Shor’s would crack it in a matter of hours (see here). This is because while today’s computers think in “bits”, a stream of electrical or optical pulses representing 1s or 0s, quantum computers use “qubits”, which are typically subatomic particles such as electrons or photons. Qubits can represent numerous possible combinations of 1 and 0 at the same time – known as “superposition” – and also create pairs that share a single quantum state – known as “entanglement”. Combined, these two properties exponentially increase their processing power and number-crunching ability (see here).
The US Department of Homeland Security estimates that quantum computers will be able to crack even our most advanced encryption systems as early as 2030 – the so-called “Q-day” –, which has slapped a 6-year confidentiality period on all encrypted data and sent governments into a race to transition to post-quantum cryptography (PQC) and other quantum-resilient encryption methods. Last month, the US Secretary of Commerce blazed a trail by approving the first standards for post-quantum cryptography (see here). Still, current estimates suggest that more than 20bn devices will need software updates, including mobile phones, laptops, desktops, servers, websites, mobile apps, and additional systems built into cars, ships, planes, and operational infrastructure (see here).
So, what exactly will quantum computing mean for copyright? Well, little research has been done on that score, but Dr James Griffin, from the University of Exeter, has been leading the way. According to his research, quantum computing could exponentially increase the number of reuses of granular elements of copyrighted works without permission, challenging our notions of fixation or fixed proprietary boundaries of protected elements. However, the interface between quantum computing and copyright is Janus-faced, with a seemingly positive side. The technology can also enhance copyright enforcement techniques, with quantum computers supporting a more fine-grained analysis of copyright infringement. Filtering mechanisms can be used to detect, prevent, and mitigate copyright infringement, and quantum watermarks can be embedded in copyrighted content to protect it from unauthorised reuse (see here).
In short, I think we can read these developments as new chapters in a familiar novel — its ending is yet to be seen, though the main thread is already known: a structural shift from an author-centred legal framework to post-factum, tentative, and ad hoc legal interventions that focus on the governance and regulation of decentralised networks rather than on the allocation of subjective rights. The embedding of opt-outs and quantum watermarks in copyrighted works streaming through all corners of the web is a de facto recognition that the economics of author-centred rights, premised on salient intertextualities, is losing ground to ever more machine-driven reuses and diffuse remixes that are humanly impossible to monitor and control. In the face of relentless technological disruption, copyright is clearly shifting from the author to the very architecture of the network. It is moving away from subjective rights towards governance-oriented legal norms, a shift that heralds a whole new era for copyright law.
_____________________________
To make sure you do not miss out on regular updates from the Kluwer Copyright Blog, please subscribe here.
Kluwer IP Law
The 2022 Future Ready Lawyer survey showed that 79% of lawyers think that the importance of legal technology will increase for next year. With Kluwer IP Law you can navigate the increasingly global practice of IP law with specialized, local and cross-border information and tools from every preferred location. Are you, as an IP professional, ready for the future?
Learn how Kluwer IP Law can support you.
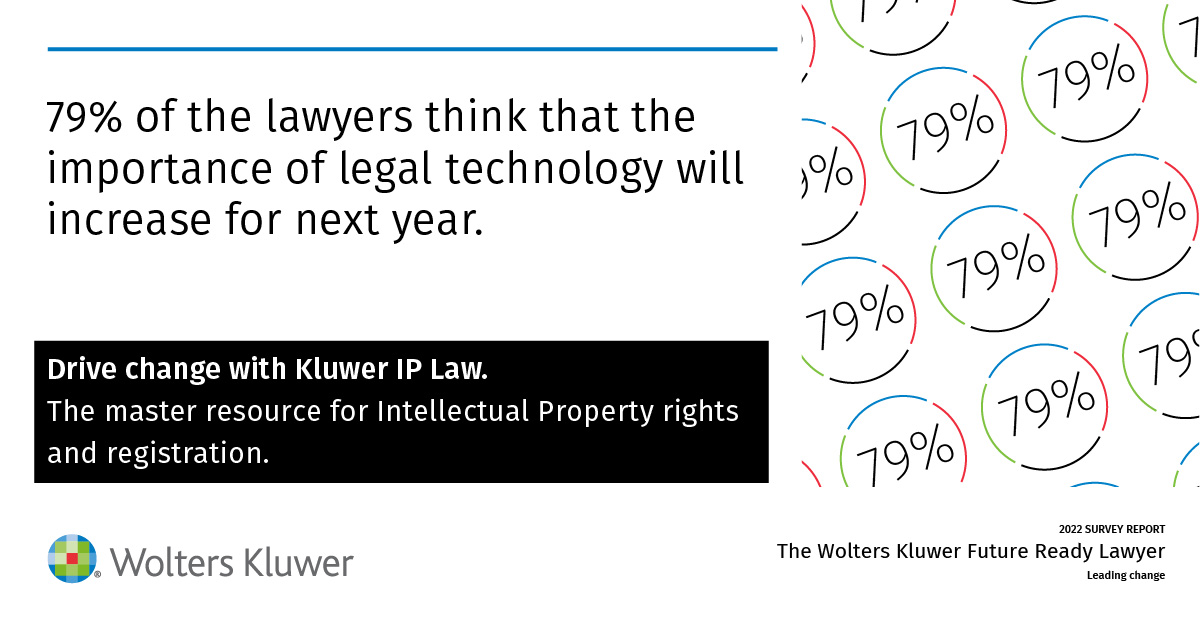
