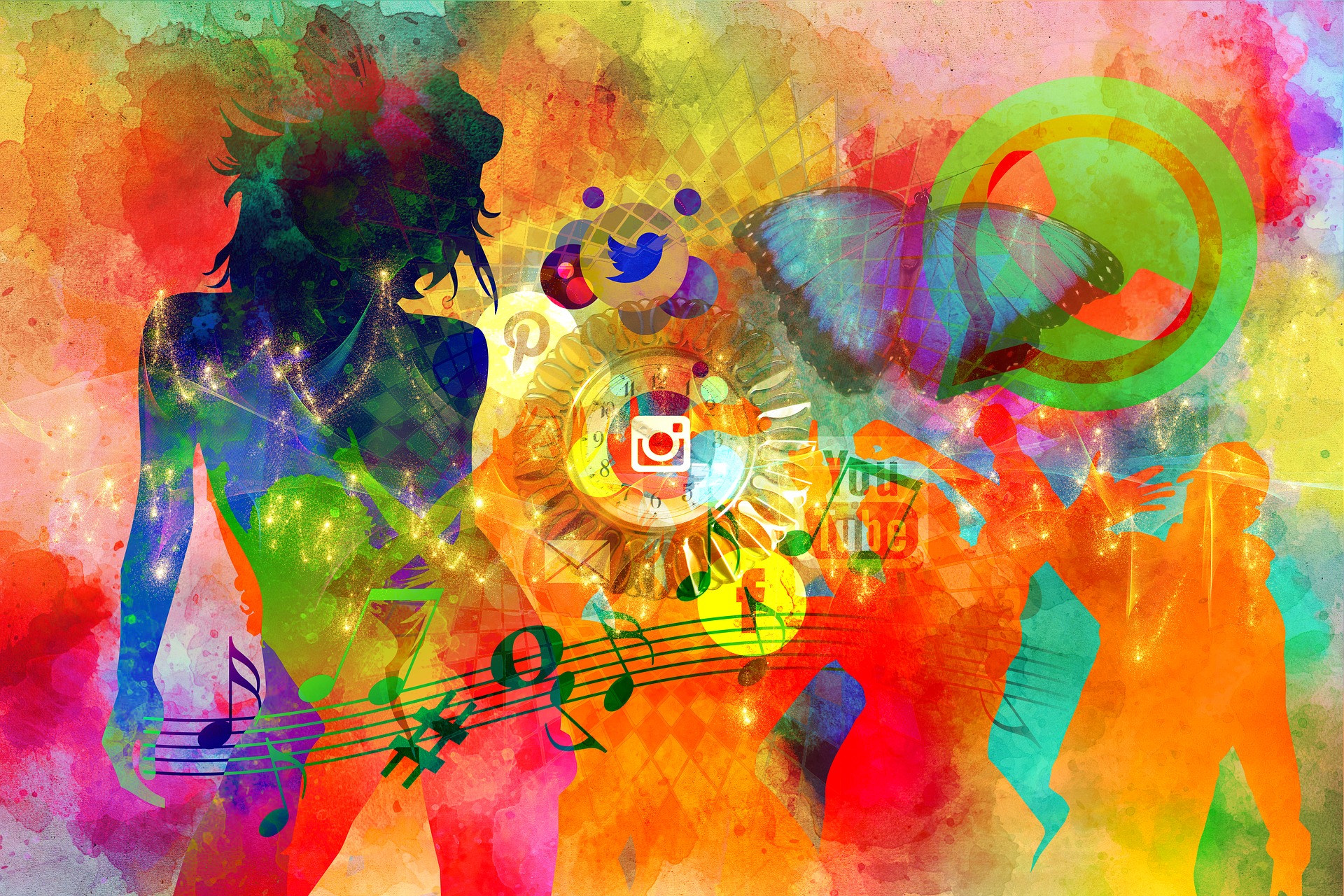
This is the second installment of a reflection on the topic of content moderation and bias mitigation measures in copyright law. The first part of this post briefly discussed the concept of bias and examined the role of property rights in data and factual information, with a focus on copyright. This second part explores the potential of property rights to increase bias in content moderation by looking at the topic from the perspective of Article 17 CDSM Directive.
Article 17, content moderation tools, and the Commission’s Guidance
Article 17 CDSM Directive regulates online content-sharing service providers (OCSSPs) through a complex set of rules. The provision states that OCSSPs carry out acts of communication to the public when they give access to works or subject matter uploaded by their users, making them directly liable for their users’ uploads. At the same time, the provision includes a liability exemption mechanism in paragraph (4), as well as several mitigation measures and safeguards in paragraphs (5) to (9).
The liability exemption mechanism in Article 17(4) encompasses a series of cumulative obligations of “best efforts” on OCSSPs to: (a) obtain an authorisation; (b) ensure unavailability of specific protected content provided they have received “relevant and necessary information” from right holders; and (c) put in place notice and take down and notice and stay down mechanisms, provided they have received a “sufficiently substantiated notice” from right holders.
In interpreting these provisions, the Commission’s Guidance (COM/2021/288 final) states that information is considered “relevant” if it is at least “accurate about the rights ownership of the particular work or subject matter in question”. The consideration of whether it is “necessary” is trickier, and it will vary depending on the technical solutions deployed by OCSSPs; in any case, such information must allow for the effective application of the providers’ solutions, where they are used (e.g., “fingerprinting” and “metadata-based solutions”).
The Guidance further states that measures deployed by OCSSPs must follow “high industry standards of professional diligence”, to be assessed especially against “available industry practices on the market” at the time, including technological solutions. When discussing current market practices that emerged from the Stakeholder Dialogues, the Guidance highlights content recognition based on fingerprinting as the main example, although recognising that is not the market standard for smaller OCSSPs. Other technologies identified include hashing, watermarking, use of metadata and keyword search; these can also be used in combination. Such technologies are sometimes developed in-house (e.g., YouTube’s ContentID or Facebook’s Rights Manager), and other times acquired from third parties (e.g., from Audible Magic or Pex). Crucially, the Guidance is a non-binding document published in the shadow of the action for annulment in Case C-401/19, and which itself recognizes that it might need revising in light of that judgment.
The CJEU’s interpretation of Article 17 and content filtering (C-401/19)
In its recent Grand Chamber judgment in Case C-401/19 (discussed here, here, here, and here), the Court clarified that Article 17(4)(b) does in fact require prior check of content by OCSSPs. In many cases, the only viable solution for platforms to moderate content is to deploy automated recognition and filtering tools, i.e., “upload filters” which constitute a justified restriction of users’ freedom of expression (see here).
The Court advances a number of arguments why Article 17 constitutes a proportionate restriction of OCSSP’s users’ freedom of expression. In essence, such arguments relate to the legislative design of Article 17 and how the provision should be interpreted and implemented in light of fundamental rights. It is also noteworthy among these arguments that the Court outlines the scope of permissible filtering. For our present purposes, the key points are the following.
- First, only filtering/blocking systems that can distinguish lawful from unlawful content are compatible with the requirements of Article 17 and strike a fair balance between competing rights and interests. Despite this important statement, establishing exactly what kind of thresholds or error rates are admissible in practice remains unclear and arguably one of the key issues in this new system.
- Second, Member States must ensure that filtering measures do not prevent the exercise of user rights to upload content that consists of quotation, criticism, review, caricature, parody or pastiche under Article 17(7). It is here where we argue that AI/ML tools will probably become essential given their superiority over fingerprinting and hashing in determining contextual uses (e.g., parody). Whether this superiority is sufficient to protect users’ fundamental rights remains an open question, but it is clear that the role played by bias and errors in this assessment is decisive.
Potential bias in copyright content moderation and error rates
So, what does the Court’s judgment mean for our discussion on bias propagation?
First, it is clear from Article 17 and the CJEU’s judgment that the only acceptable point of reference to deploy (re)upload filters is the information and/or notice provided by right holders under Article 17(4)(b) and (c). Depending on the technology employed, the information provided will play a critical role in the correct identification of the allegedly infringing material. A dedicated analysis should be carried out for each of the two main groups of content moderation approaches adopted in this field: matching (fingerprinting, hashing, metadata, etc.) and predictive analysis (AI/ML). It suffices here to say that the process of identification of the input data and its use to classify users’ uploaded content is liable to embed the potential bias and errors identified in the first part of this blog. This bias may very well influence the ability to correctly identify content (false positives and/or negatives), the ability to establish acceptable thresholds (e.g., a 20% match, a 90% match), and, most importantly for the analysis here developed, the (in)ability to correctly determine contextual uses in order to safeguard users’ fundamental rights as provided for in Article 17(7).
Second, such filters must be able to distinguish lawful from unlawful content without the need for an independent assessment by the providers. As major platforms have admitted during the stakeholder dialogues, current moderation tools are not capable of assessing these contextual uses. That suggests both a future push towards a more AI/ML intensive approach but also explains the current dependence of existing filters on error thresholds to distinguish between what content is blocked and what content stays up.
According to the above, the core question may be reformulated as a question about what type of errors or bias are legally acceptable. The Advocate General (AG) in his Opinion considered it crucial to ensure that the error rate of “false positives” resulting from the deployment of content recognition tools by OCSSPs “should be as low as possible”. Hence, where “it is not possible, in the current state of technology… to use an automatic filtering tool without resulting in a ‘false positive’ rate that is significant, the use of such a tool should… be precluded” [para 214]. In the AG’s view, allowing ex ante filtering in such cases of ‘transformative’ content would “risk causing ‘irreparable’ damage to freedom of expression” [para 216]. Although the Court was less detailed than the AG, it generally endorsed this approach of preventing overblocking and the risk of chilling freedom of expression by focusing on avoiding false positives.
In spite of the above, the question as to what degree of error is acceptable and how to ensure unbiased results remains largely answered. The most difficult step may well be the design and training of algorithms able to assess potentially complex copyright law questions, e.g., under what conditions a certain use should be classified as parody or criticism. On the one hand, it seems almost impossible to encapsulate the concept of parody or criticism in an error-rate percentage. On the other hand, when ML algorithms are deployed instead, it appears similarly dubious that the priority followed in selecting training data was to allow filters to replicate legally educated answers. As we have seen in Part I, the incentives currently driving this market, especially in the EU, point towards other priorities, such as cost reductions, legal certainty and in-house confidential development. Consequently, if the scenarios introduced in Part I are plausible, parties involved or affected by algorithmic content moderation will need to familiarise themselves with questions in cluding how a US model trained for parody detection may influence error rates in the EU market; or how algorithms trained on large language corpi or pop music would perform on smaller languages, or niche repertoires. In the light of the CJEU’s judgment, questions like these remain open. Delegating market or industry dynamics to offer answers carries the risk of denying effective protection to user rights in Article 17(7).
Conclusions: looking forward
It seems that –at least prima facie– property rights in data may possess the unexpected effect of favoring errors and bias propagation into the trained AI. This effect seems noticeable in areas where AI is already deployed such as in the context of content recommendation, but it may be expected to also become more relevant in algorithmic content moderation, especially with the increasing adoption of ML approaches.
As argued, bias propagation does not follow a unique pattern, but may develop along different lines depending on the type of technology employed and how this technology relates to input data. The general principles and the arrival point, however, appear to be similar. A reduced availability and transparency of input/training data has a negative effect on access, verification and replication of results, which, in turn, are ideal conditions for bias and errors to the detriment of users’ rights.
What mechanisms or remedies can we envision to mitigate the propagation of bias from data to AI? Can they be found within the field of property rights? Several approaches may be conceived:
- Internationally, a broad call for user rights to research in AI has been proposed, which would enhance data retrieval from protected works. This would enhance access to relevant data (especially training data) and consequently favor a more open, transparent and verifiable data ecosystem.
- In legal systems relying on open standards such as the US, fair use has been identified by some authors as a powerful bias mitigation device (Levendowski 2018). At the same time, other authors have pointed out the risk that algorithmic enforcement systems deployed by large-scale platforms like YouTube (ContentID) or Meta (Rights Manager) may ultimately “become embedded in public behaviour and consciousness”, and thus progressively shape the legal standard itself, by habituating the “participants to its own biases and so progressively altering the fair use standard it attempts to embody” (Burke 2017).
- This same risk is clear for the algorithmic content moderation systems of large-scale OCSSPs under Article 17 CDSM (some of which will qualify as “very large online platforms” under the Digital Services Act). Such large platforms have the power through their algorithms to crystallize the cultural and ultimately legal meaning in the online environment of the concepts underlying the E&Ls for quotation, criticism, review, caricature, parody or pastiche.
- From a legal design perspective, an adversarial procedure has been proposed, i.e. the ability to contest algorithm determinations by introducing a public adversarial AI which embeds counterbalancing values (Elkin-Koren, 2020). In this way, users and the public at large may be empowered in their challenges against algorithms that are designed by platforms in collaborations with right holders.
- In the EU, it could be interesting to explore to what extent AI applications employed for content moderation could or should be considered as high-risk AI systems in the sense proposed by the AI Act. As stated in the AI Act proposal, “for high-risk AI systems, the requirements of high-quality data, documentation and traceability, transparency, human oversight, accuracy and robustness, are strictly necessary to mitigate the risks to fundamental rights”. Whereas this solution certainly needs further consideration, it possesses several of the elements necessary to mitigate the discussed perils of bias propagation in content moderation.
Algorithmic content moderation is a powerful tool that may contribute to a fairer use of copyright material online. However, it may also embed most of the bias, errors and inaccuracies that characterize the information it has been trained on. Therefore, if the user rights contained in Article 17(7) CDSM Directive are to be given an effective protection, simply indicating the expected results omitting how to reach them, may not be sufficient. The problem of over-blocking is not simply a technical or technological issue. It is a cultural, social and economic issue, as well and, perhaps more than anything, it is a power dynamic issue. It is unrealistic to put on equal footing the threat of a (primary) copyright infringement action brought by right holders due to under-blocking on the one hand, with that of individual users experiencing the removal of their parody or criticisms on the other, especially considering that users normally agree in the terms of service of platforms that blocking of their content is at the sole discretion of the platform. Recognizing parody, criticisms and review as “user rights”, as the CJEU does in C-401/19, may be a first step towards the strengthening of users’ prerogatives. But the road to reach a situation of power symmetry with platforms and right holders seems a long one. Ensuring that bias and errors concealed in technological opacity do not circumvent such recognition and render Article 17(7) ineffective in practice would be a logical second step.
Acknowledgments: This research is part of the following projects. All authors: the reCreating Europe project, which has received funding from the European Union’s Horizon 2020 research and innovation programme under grant agreement No. 870626. João Pedro Quintais: VENI Project “Responsible Algorithms: How to Safeguard Freedom of Expression Online” funded by the Dutch Research Council (grant number: VI.Veni.201R.036).
This blog post is based on the EPIP2021 roundtable organised in Madrid (September 8-10, 2021). The authors are grateful to Prof. Niva Elkin-Koren and to Dr. Irene Roche-Laguna for their participation and for their insightful perspectives and suggestions which have been helpful in developing this analysis. The blog post only reflects the view of the authors and any errors remain our own.
________________________
To make sure you do not miss out on regular updates from the Kluwer Copyright Blog, please subscribe here.
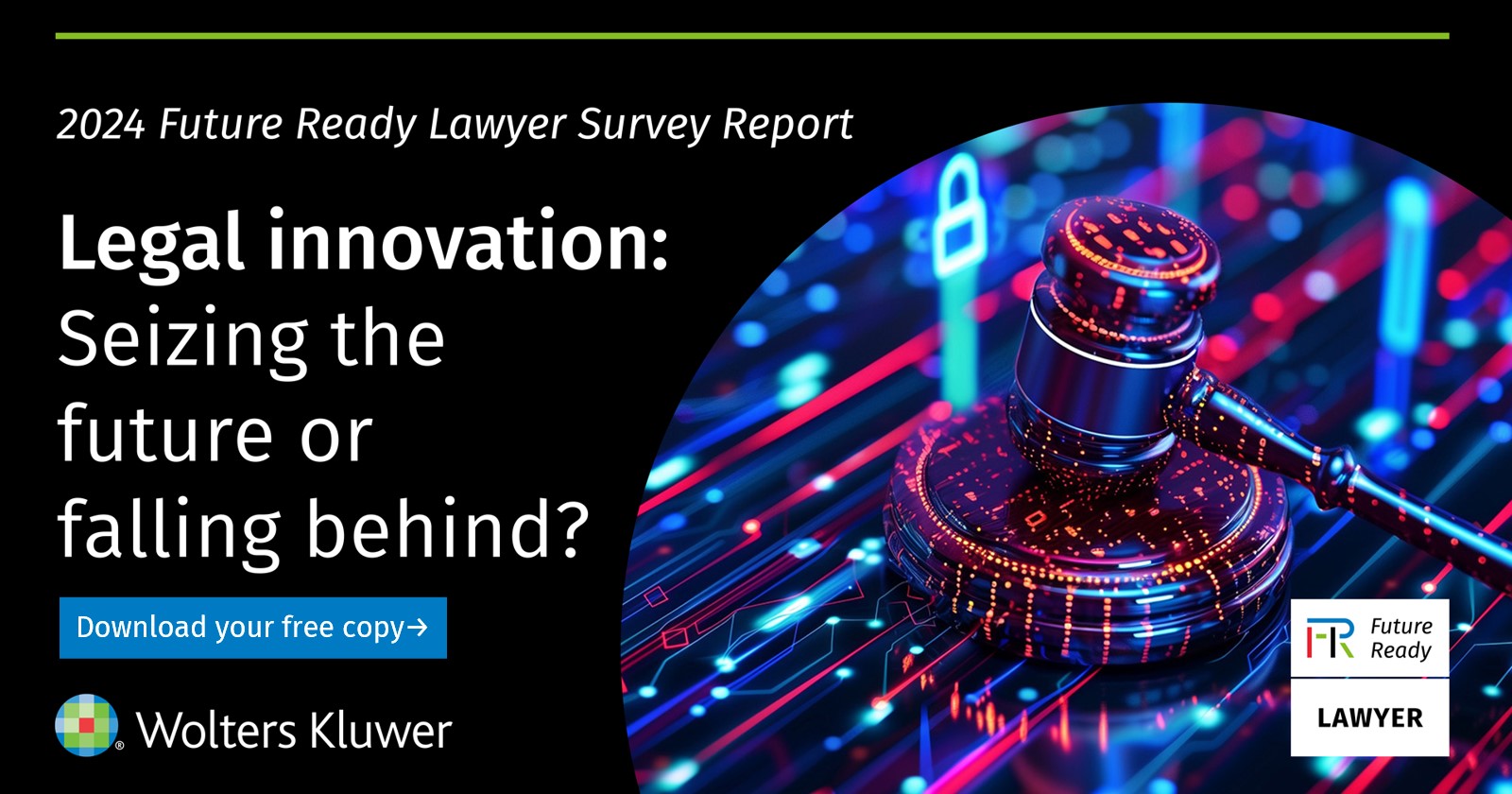
