As generative machine learning (ML) systems become more mainstream, the discussion about copyright and ML input is back in the spotlight. At the heart of this discussion is the question of whether authors, creators, and other rightholders need to give permission before their works can be used as input for generative ML systems that produce outputs based on the works on which they have been trained.
The issue is beginning to be litigated in the United States and the United Kingdom. There are currently at least three lawsuits (two in the US (see here and here) and one in the UK (see here)) alleging that training generative ML models on publicly available works is copyright infringement. All three lawsuits name Stability AI, the developer of the open-source image generator Stable Diffusion, as a defendant.
Meanwhile, we have also seen calls from organizations representing creators for explicit legal protection against unauthorized use of works for ML training. Such calls have emerged in the US and in the EU, where they have been raised in the context of debates on the proposed AI law currently being considered by the EU legislature. According to press reports, “artist associations are mobilizing to introduce a specific section in the Act dedicated to the creative arts, including safeguards requiring that rightholders give explicit informed consent before their work is used.”
So what is the current legal situation regarding the use of publicly available copyrighted works for the purpose of training ML systems?
In the US, this is largely up in the air, but the above-mentioned lawsuits are expected to shed some light on whether such uses should be considered “fair use” (as claimed by the developers of the ML systems in question), or whether they require explicit permission from rightholders.
In Europe, the legal framework is much clearer (which probably explains why all of the current lawsuits have been filed outside the EU). This is because, since the adoption of the Copyright in the Digital Single Market (CDSM) Directive in 2019, the European Union has harmonized the rules that apply to the use of copyrighted works for training ML systems.
ML = TDM
Articles 3 and 4 of the Directive introduce a set of exceptions to copyright for so-called text and data mining (TDM). And while the terminology used here does not immediately evoke discussions of machine learning and artificial intelligence, it clearly applies to the issue at the heart of the current debate, as further explained below. In fact, the discussion about TDM during the legislative battle over the Copyright Directive has always been about the ML revolution that was already on the horizon at the time[1].
The CDSM Directive defines text and data mining as “any automated analytical technique aimed at analyzing text and data in digital form in order to generate information which includes but is not limited to patterns, trends and correlations.” This definition clearly covers current approaches to machine learning that rely heavily on correlations between observed characteristics of training data. The use of copyrighted works as part of the training data is exactly the type of use that was foreseen when the TDM exception was drafted[2].
While the exception in Article 3 of the Directive allows text and data mining for the purposes of scientific research by research organizations and cultural heritage institutions, as long as they have lawful access to the works to be mined, it is the exception in Article 4 of the Directive that is most relevant to the current discussion.
The Article 4 exception – which is the result of extensive advocacy by researchers, research organizations, open access advocates and technology companies to broaden the scope of the TDM exception in Article 3[3] – allows anyone to use “lawfully accessible works” for text and data mining unless such use has been “expressly reserved by their rightholders in an appropriate manner, such as machine-readable means.”
Taken together, these two articles provide a clear legal framework for the use of copyrighted works as input data for ML training in the EU: researchers at academic research institutions and cultural heritage institutions are free to use all lawfully accessible works (i.e., the entire public Internet) to train ML applications. Everyone else (including commercial ML developers) can only use works that are lawfully accessible and where the rightholders have not explicitly reserved use for text and data mining purposes.
To adherents of the ML learning = innovation = economic growth narrative, this framework, which gives creators and other rightholders the ability to opt out of – or, more likely, demand compensation for – the use of their works by commercial ML developers, will feel like a significant restriction that is innovation-hostile. And as long as the presumption remains that in other parts of the world, the use of copyrighted works for ML training constitutes “fair use,” they will see it as a significant competitive disadvantage for the EU economy[4].
New forms of collective action?
Many creators have a very different reaction to the emergence of generative ML systems. They worry that their creative work will be exploited by companies building generative ML applications, which in turn will weaken the demand for their work. There are concerns that all the value generated by these tools will go to large tech companies, with none going to the artists and creators whose work is used to train these models. Others are concerned about the commodification of their unique artistic styles[5], or simply want to control how and by whom their work is used. For all of them, the EU approach to TDM /ML opens up an interesting perspective: They can use their ability to opt-out as leverage to set terms and demand compensation.
Given the scale of ML training (which for foundational models is at the scale of the Internet), this lever is unlikely to work very well if used by individual creators on their own. Instead, it seems clear that creators will need to band together to collectively enforce their rights against those who wish to use their works as input for ML training.
This means that there is a huge opportunity for creators to build new[6] collective structures for exercising their rights vis-à-vis commercial ML developers. This is an opportunity for artists, authors, and other creators to build digitally native forms of collective organization that rely on open protocols to communicate their norms and terms. Perhaps most importantly, it is also an opportunity to band together as creators and demand a seat at the table when it comes to developing the norms and practices that will shape artistic production in a world of ubiquitous generative ML systems.
We are already seeing early attempts to do this, such as Mat Dryhurst and Holly Herndon’s spawning.ai initiative, which is in the process of developing a toolset for opting out of ML training. Herndon and Dryhurst situate their approach between the “polar ideologies of free culture or rigid IP protectionism of the last century”, both of which they consider “insufficient for tackling an issue that promises to mutate into a long culture war.”
While it is largely unclear at this point what such a third way of regulating the use of copyrighted works might look like, it is clear that it will need to be shaped by the creators whose works are used to train ML systems and who also use the ML-powered tools as part of their creative processes.
Solutions are likely to resemble direct remuneration rights derived from the revenues generated by trained models[7], managed collectively by artists and creators, bypassing the services of traditional CMOs and other intermediary-type rightholders such as publishers.
The future of copyright?
And while the EU legal framework for TDM/ML appears to be more restrictive than those outside the EU (at least until questions around fair use are settled) and has been perceived as a loss by many in the research and access to knowledge community who have argued for a more open approach based on the principle that “the right to read is the right to mine,” the mechanism in Article 4 points to a future for copyright that is much better adapted to the realities of the digital environment.
Instead of a blanket extension of copyright to all forms of text and data mining outside the academic context (as originally proposed by the Commission), the EU legislator has ensured that in the context of TDM/ML, copyright protection will only accrue to those creators and rightholders who actually want it enough to signal their intent. This approach addresses one of the most fundamental problems with copyright: that it applies by default to all creative output — both by creators who wish to control the use of their works and by those who do not. The EU framework for TDM limits copyright protection to those creators who want it, without covering the rest of human expression on the Internet with the suffocating blanket of default copyright protection that would lock those works away for many decades.
For now, this opt-in approach to copyright is limited to TDM, but it is not inconceivable that this approach could be expanded if it proves to work in practice, especially in the ongoing discussion about ML training.
To anyone interested in building a more modern EU copyright framework that moves towards a registration-based approach, this should be yet another reason why it is important for artists, authors, and other creators to band together and use the tools that the EU legislator has given them instead of asking for an unnecessary and likely counterproductive further expansion of copyright.
This post was first published on the Open Future blog.
[1] The European Parliament’s summary published after the adoption of the Directive makes this explicit by noting that “the co-legislators agreed to enshrine in EU law another mandatory exception for general text and data mining (Article 4) in order to contribute to the development of data analytics and artificial intelligence”
[2] This analysis is based on the generally accepted understanding that trained ML models do not contain copies of the works that they have been trained on. While there are studies that show that in some cases diffusion models can “memorize” works contained in their training data this seems to be an extreme outlier.
[3] This statement by 24 stakeholders stresses “the foundational role that TDM plays in Artificial Intelligence (AI)”
[4] In this context it is interesting to note that the other notable non-EU competitor of the EU — the United Kingdom — has just abandoned efforts to introduce a TDM exception that would also cover uses in commercial contexts under considerable pressure from rightholders.
[5] There is also work being done on tools that “Protect Artists From A.I.-Generated Art That Steals Their Style”
[6] This opportunity also exists for traditional collective management organizations, although it seems that they are too slow, too old, too territorial, too technology averse and too expression-specific to address this challenge in a timely fashion.
[7] Given the apparent parallels of the current phase of ML development with previous phases of original accumulation it might make even more sense to trade permission in return for equity.
________________________
To make sure you do not miss out on regular updates from the Kluwer Copyright Blog, please subscribe here.
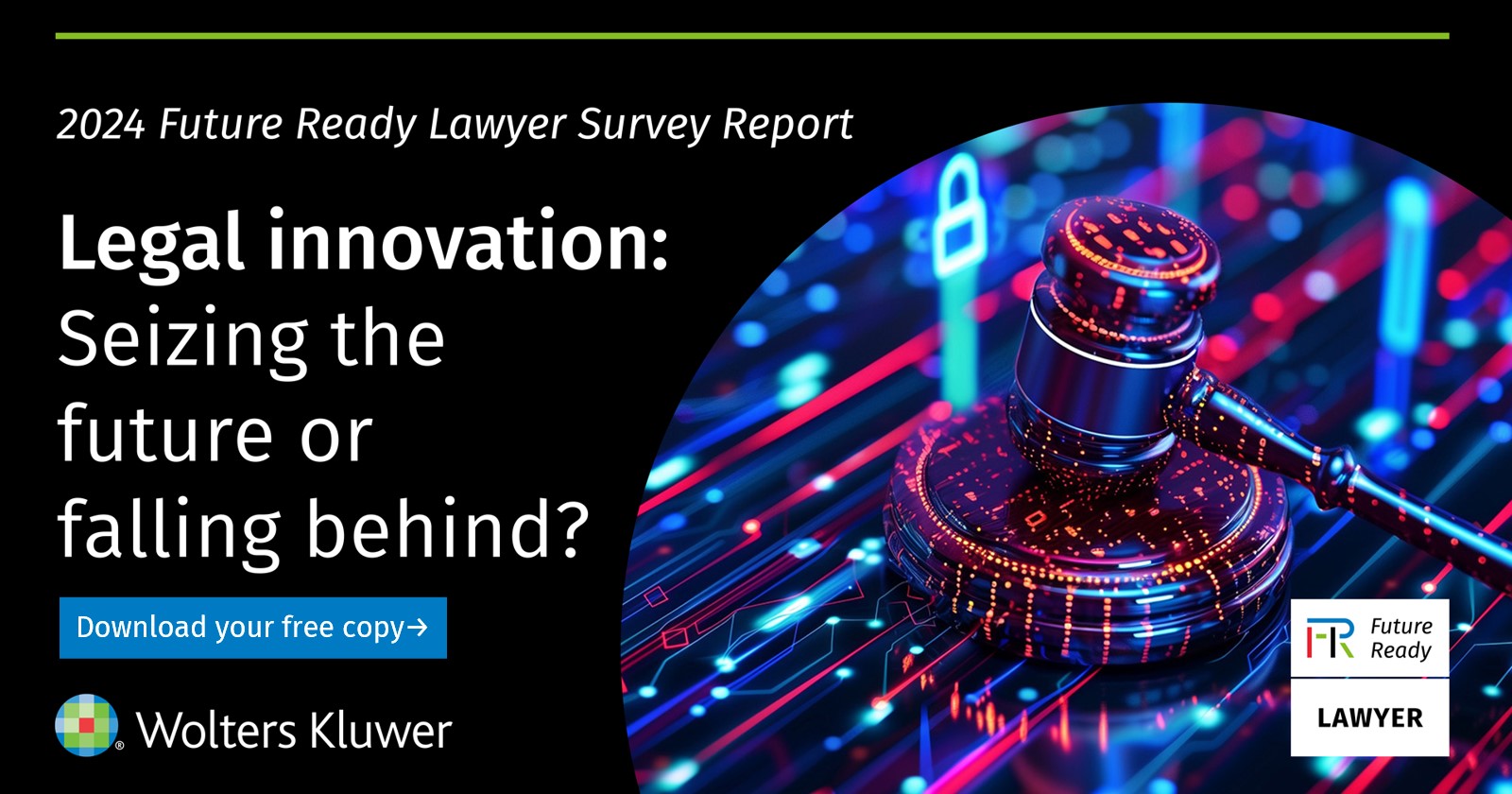

Sometimes I find the articles on this website too technical and difficult to understand for laypeople. Maybe it could be simplified or added with more detailed explanations.