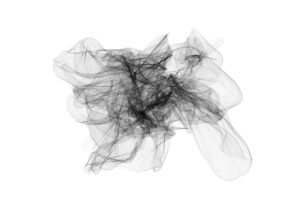
The sweeping evolution of generative AI models is rapidly reshaping the legal landscape of copyright. In the wake of the landmark cases of Authors Guild, Inc v HathiTrust and Authors Guild, Inc v Google, Inc – or the Google Books case –, the fair use doctrine has accommodated a core principle of non-expressive use, referring to any act of reproduction that is not intended to enable human enjoyment, appreciation, or comprehension of the copied expression (see here). While the principle is premised on the age-old idea-expression dichotomy, whose roots stretch right back to the beginnings of copyright, it is today what allows one to distinguish the expressive work from the meta-level information – facts, ideas, indexes, statistics, trends, correlations – that can be extracted from that work without infringing potential copyright. To put it more metaphorically, it is the legal green light for web crawlers nowadays to scour all corners of the internet, scraping information from websites and databases, indexing their content, and storing it for later retrieval, typically by search engines. Copy-reliant technologies have banked heavily on that principle over recent years and it wouldn’t be a stretch to say that the principle of non-expressive use has become the legal foundation of how the internet essentially works.
But the rapid spread of generative AI models, the latest evolution of copy-reliant technology, has posed another set of challenges to copyright. Litigation against these models has piled up at the same breakneck speed as they have gained ground. And at the core of this litigation lies a common claim: generative AI has a memory problem. This is an important shift from past litigation involving copy-reliant technologies and therefore merits a fresh look. So, what does this memory problem actually mean? In machine learning, the inherent trade-off between memorisation and generalisation is one of the “known unknowns” of the trade. It is still unknown because machine learning experts are still grappling with this conundrum in the hopes of coming up with the best solution for striking the right balance between the two.
Memorisation is a machine learning phenomenon closely bound up with what is known in the trade as “overfitting” (see here and here), and has been observed in transformers and diffusion models alike (in the case of diffusion models, see, for instance, Getty Images, Inc v Stability AI, Inc; and in the case of transformer-based models, see here). It means that the model memorises the training set more than it should; it ‘fits’ the training set so well that it is unable to generalise, or – which comes to the same thing – project its stochastic predictions onto fresh, unseen data. In other words, a model with a memory problem is prone to inadvertently reveal pieces of the original training set if properly nudged with specific prompts, thus crossing the threshold of ‘reproduction’ or ‘substantial similarity’ between the copyrighted works used in the training set and the output generated by the model. It’s like Funes, el memorioso, the main character of a short tale written by Jorge Luis Borges, who was able to remember every day of his life down to the tiniest detail, but who was a fool at heart, utterly incapable of understanding, generalisations, or abstractions.
Several possible causes of overfitting have been reported in the literature: high complexity of the AI model, leading it to mould too closely to the training data; limited training data; and too much noisy data, affecting the model’s ability to distinguish relevant information – a signal – from the irrelevant – a noise. The computer science literature suggests, for instance, that memorisation is more likely when models are trained on many duplicates of the same work. This explains why it is easier to prompt a model to infringe copyrightable characters with a strong visual component and media ubiquity, such as Snoopy, than to infringe a Salvador Dalí painting (see here).
Underlying all cases of robotic reading, whether in search engines or generative AI, are basic computational processes that apply structure to unstructured electronic texts and employ statistical methods to lay bare new bits of meta information and reveal latent features inherent in the processed data. This has been commonly referred to as TDM or “text and data mining”, one of the building blocks of machine learning and internet search technology. In the EU, TDM activities have relied on explicit exempting provisions enshrined in the Directive on Copyright in the Digital Single Market (CDSMD). Of particular concern is the so-called commercial exception in Art. 4 CDSMD – incorporated e.g. into the German Copyright Act under Section 44b –, which provides that reproductions and extractions may be retained for as long as necessary for the purpose of text and data mining on condition that the use of works has not been expressly reserved by the rightholder by machine-readable means. Effectively, the provision established an “opt-out” mechanism for copyright holders to reserve their copyright.
In an ever more fragmented digital landscape, this provision has become a key instrument of self-regulation, playing a crucial role in the allocation of rights and obligations around the licensing of copyrighted works as training data (see here). By April last year, over one billion pieces of artwork had been removed from the Stable Diffusion training set. But for all the technical preparation of certain websites and organisations to effectively opt-out in a machine-readable format, a lingering question has always been whether generative AI models are technically prepared to read these machine-readable opt-outs; moreover, how to ensure that they respect these opt-outs? And if they fail to observe the opt-outs, how can copyright holders know whether their copyright has been infringed?
This is where the AI Act comes in. There are at least two provisions that merit attention, as they mark a welcome step in the right direction. Article 53(1)(c) sets out the obligation for general-purpose AI model providers to put in place a copyright compliance regime, i.e. a policy to respect Union copyright law, in particular to identify and respect, including through state-of-the-art technologies, the reservation of rights expressed under Art. 4(3) CDSM. And Article 53(1)(d) imposes an additional obligation on providers of general-purpose AI models to create and make publicly available a sufficiently detailed summary of the content used in the training of the model – according to a template to be provided by the AI office. Together, these two provisions technically facilitate the exercise of opt-outs and shift more allocative power to copyright holders (see here). According to Recital 107, while due account should be taken of the need to protect trade secrets and confidential business information, the summary is to be generally comprehensive in its scope to facilitate parties with legitimate interests, including copyright holders, to exercise and enforce their rights under Union law, for example by listing the main data collections or sets that went into training the model, such as large private or public databases or data archives, and by providing a narrative explanation about other data sources used.
Scarcely a day goes by without news of exciting breakthroughs in the world of AI. In the face of disruptive waves of technological change and mounting uncertainty, the law cannot help but take on an “experimental” character, with lawmakers and lawyers often caught on the back foot, struggling to keep up with the sweeping winds of change. But whatever the next steps may be, one thing is certain: litigation surrounding generative AI marks an important crossroads, and whichever path we choose is likely to shape the future of the technology. The rising litigation around generative AI is not targeting image by image or specific excerpts of infringing texts produced by AI models. Rather, the whole technique behind the system is hanging in the balance.
Another key takeaway that merits attention relates to the fragmentary landscape of copyright that seems to be unfolding in the wake of the rapid advances in AI technology. Although the emerging European legal framework offers strict rules yet solid ground for AI technology to flourish on the continent, it’s worth wondering what will happen if the “Brussels effect” fails to reach the shores the other side of the Atlantic and the use of copyrighted works for training purposes is found to be transformative fair use in common law jurisdictions, while a relevant portion of these works are opted-out of AI models on European soil. That would mark a yawning gap between two copyright regimes, opening a new chapter in this old tale and potentially disadvantaging would-be European generative AI providers.
_____________________________
To make sure you do not miss out on regular updates from the Kluwer Copyright Blog, please subscribe here.
Kluwer IP Law
The 2022 Future Ready Lawyer survey showed that 79% of lawyers think that the importance of legal technology will increase for next year. With Kluwer IP Law you can navigate the increasingly global practice of IP law with specialized, local and cross-border information and tools from every preferred location. Are you, as an IP professional, ready for the future?
Learn how Kluwer IP Law can support you.
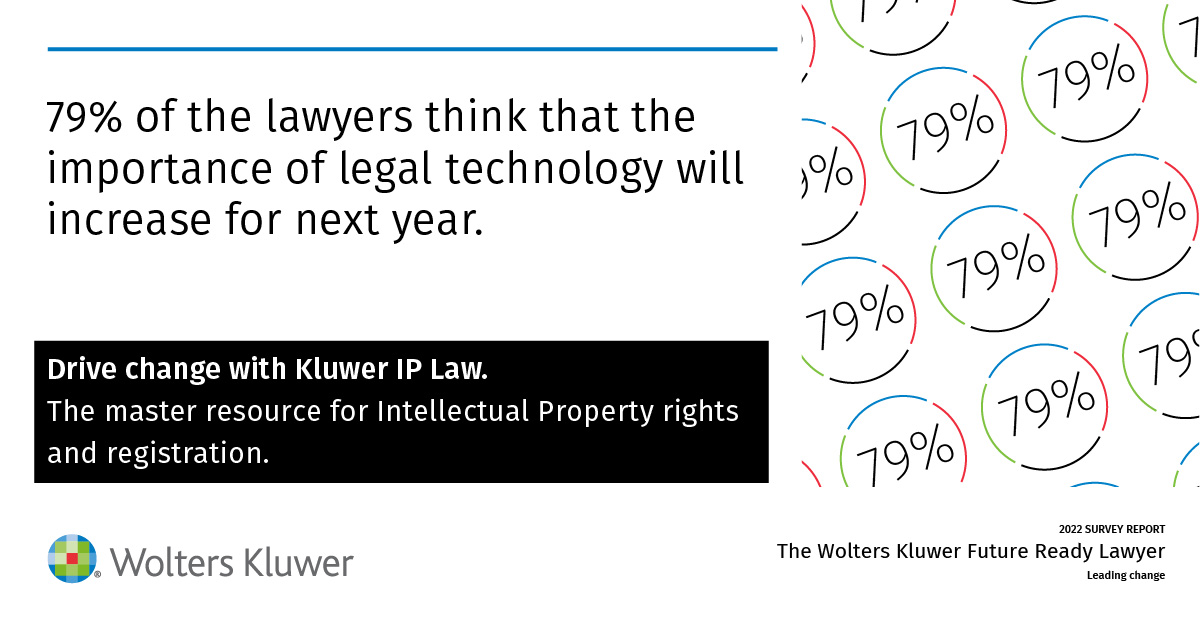

As a layman, with no background in the digital arts, but with a considerable interest in AI, I found this exposition and discussion accessible and informative. Thanks for this, and also for the opportunity to congratulate you. Cheers!