A series of recent amendments to copyright law, including in the EU Copyright and the Digital Single Market Directive (Art. 3 and 4) and in Singapore’s new Copyright Act (Art. 243, 244), seek to protect the ability of text and data mining researchers to use copyrighted content in their work. “Text and data mining” (“TDM”) describes any application of a computational process to materials to derive data from or about those works. TDM can be used to help train computer applications to engage in machine learning or artificial intelligence (“AI”) which applies additional analysis and processes to enable machines to dynamically “learn” new tasks for which they were not specifically programmed. An extensive study of research exceptions in over 190 countries shows that copyright may be a hindrance to certain kinds of TDM research in most of the countries of the world today. These examples help illustrate the broad public benefits that can accrue from harmonizing copyright exceptions for research uses in the digital environment.
Speeding literature review
One of the most common uses of TDM is to help scholars find, read, and analyze information in academic journals and other sources. For instance, a study by Zhen Wang et al studied the error rates of human reviewers during abstract screening in systematic reviews and suggested false inclusion and exclusion rates by human reviewers. Similarly, a study by Candyce Hamel et al showed how crucial AI and active machine learning can ease future medical research by title and abstract screening. In the same vein, studies by Piotr Przybyła et al and Alison O’Mara-Eves et al show the usefulness of AI and data mining for prioritizing and identification in Systematic Reviews. With the decisions in the USA and legal changes in other countries permitting greater use of TDM without additional licensing of the underlying works, a number of research tools have become available to aid automated literature review, including EvidenceFinder, ASReview, Covidence, DistillerSR (see this user study on DistillerAI), JBI SUMARI, Colandr, Rayyan (see also here), RobotAnalyst, Anni. Many of the projects described below use this basic function of TDM in various specific applications. Studies have shown significant improvement in results from TDM research using full-text articles, which are often behind a paywall or subject to additional licensing, rather than mere abstracts, which are often more freely available. For example, David Westergaard et al analyzed 15 million full-text articles in English from 1823-2016 and compared the findings to results obtained from 16.5 million abstracts. The results revealed that using text mining on full-text articles consistently yielded better results compared to using abstracts alone.
Enabling medical discovery
Medical researchers often use TDM to investigate new uses of medicines and other treatments that can lead to important breakthroughs. For example, text mining research helped identify uses of thalidomide, a drug that had been removed from the market, to treat chronic hepatitis, and helped discover a new link between genes and osteoporosis that led to new treatment protocols.
Epidemic and Pandemic Tracking
The outbreak of a novel coronavirus from Wuhan, China, later named COVID-19, was first discovered by a Canadian artificial intelligence firm called BlueDot. The firm analyzed “a variety of information sources, including chomping through 100,000 news reports in 65 languages a day” to recognize patterns between health outbreaks and travel (see also here). Other TDM projects have examined social media and other online sources to track and explain COVID-19 vaccination hesitancy, and to identify High-Risk COVID-19 patients. For example, Shasha Teng et al analyzed a dataset of 43,203 YouTube comments to scrutinize the correlations between vaccine hesitancy factors and vaccination intention. Medical Home Network (MHN) used TDM methods in predictive health risk screening to identify Medicaid patients who are at the highest risk of COVID-19.
Vaccine Research
COVID-19 research benefited from TDM projects that mined scientific publications about the coronavirus family, helping to speed the identification of vaccine candidates. Illustratively, see Hao Lv et al’s study “present[ing] an extensive survey on the application of AI and ML for combating COVID-19 based on the rapidly emerging literature,” AlphaFold’s computational predictions of protein structures associated with COVID-19, and Xu Li et al’s study analysing “the genome sequence of SARS-CoV-2 and identified SARS as the closest disease, based on genome similarity between both causal viruses, followed by MERS and other human coronavirus diseases”. Likewise, A. S. Albahri et al’s study titled “Role of biological Data Mining and Machine Learning Techniques in Detecting and Diagnosing the Novel Coronavirus (COVID-19): A Systematic Review” confirms that “data mining and ML techniques in medical fields can provide the right environment for change and improvement”.
Identifying Disinformation and Hate Speech in Media
TDM researchers tracking and exposing disinformation need to make and share reproductions of copyrighted media, including news reports, blogs, websites, social media, and other sources. Examples include GoodNews, which aims to “build the technological capability for algorithmic fake news detection in social media”, and FANDANGO, which strives “to aggregate and verify different typologies of news data, media sources, social media, open data, so as to detect fake news and provide a more efficient and verified communication for all European citizens”. TDM has been used in several efforts to combat misinformation about COVID-19, including by researchers at UC Riverside, in the United States (see their paper here), and by Xuehua Han et al in China (analyzing Sina-Weibo, a Twitter-like microblogging system).
Decolonizing Language Translation Tools
Training language translation tools require a large corpora of text written in the languages being translate from and to. Obtaining sufficiently large amounts of text can be difficult even for well-resourced languages such as English, French and Spanish. One researcher explained:
“The main issue really is clarity. We often discuss if we can just crawl the web to create very large corpora of linguistic data. There’s a lot of uncertainty from our side in terms of to what extent that would be allowed. That’s why we focus on established data sets but it would be a great boost to language understanding research to leverage the data in huge web corpora. If we knew specifically how far we are allowed to go when crawling data and using it for research, this would be very helpful.”
The problems with creating adequately large training data sets are compounded for “low resource languages” in Africa and elsewhere. The Masakhane, project, for example, seeks to “spur [natural language processing” research in African languages, for Africans, by Africans.” Masakhane projects in South Africa and Kenya, for example, are building translation tools that can translate academic articles into Swahili, Zulu and other indigenous languages in an effort to “decolonise science.” But training these tools requires the ability to reproduce and mine newspaper articles and other texts written in African languages, which some publishers have refused permission to use. The project is therefore studying their rights to make unauthorized research uses under African copyright law.
Examining Gender in Literature
A study on the Transformation of Gender examined a collection of over 100,000 novels in the HathiTrust Digital Library collection from 1703 to 2009. It analysed the differences in language used to discuss male-identified and female-identified fictional characters, finding that from the nineteenth century through to the early 1960s, the proportion of female-identified character space decreased. The study was made possible through the reproductions of books by the Google Books project and provided to the HathiTrust, whose making available of the resource for text and data mining research was held to be a fair use in Authors Guild v. HathiTrust, 755 F.3d 87 (2d Cir. 2014).
Learning analytics to improve educational policies in Uruguay
The educational authorities of Uruguay have signed a contract with a well-known company that provides virtual classroom services for the Primary and Secondary levels of public and private education. But the terms of use of the platform do not allow text and data mining research and Uruguay’s law does not provide an applicable exception. This lack of clear legal authority has dissuaded the National Research Agency of Uruguay from using learning platform data in its project to create “Prediction models for the determination of academic risk, which seeks to create an early warning system for academic risk in public primary and secondary education students in Uruguay.
Inadequacy of Restricting Research to Open Access Sources
Due to concerns about copyright, TDM researcher often restrict their uses to materials published under open-access copyright licenses. But limiting training data to open access sources may create various forms of bias in research results. Many language training models, for example, limit their training data to Wikipedia articles. But English language articles dominate Wikipedia pages and many languages, such as Ndebele — an official language in South Africa, have no Wikipedia pages at all. Many critical research articles needed in TDM are not published open access. For example, only 62% of the UK PubMed Central articles on malaria are open to text and data mining research.
Conclusion: Toward a right to research in international copyright
To promote the greatest possible use of technology in scientific research, academics (including in a recent opinion published in Science), and a new “Access to Knowledge Coalition,” are calling for countries to work together, including in international forums, to promote the extension of research exceptions into the digital environment. The above examples of TDM research illustrate some research activities that can be undertaken where “open” research exceptions exist (as that term is described by Flynn and Palmedo), but that can be thwarted in more closed systems (see Flynn et al). All countries should review their laws and clarify their application in the digital sphere and support international efforts, such as at the World Intellectual Property Organization and UNESCO, to prevent copyright from being a barrier to research and science.
_____________________________
To make sure you do not miss out on regular updates from the Kluwer Copyright Blog, please subscribe here.
Kluwer IP Law
The 2022 Future Ready Lawyer survey showed that 79% of lawyers think that the importance of legal technology will increase for next year. With Kluwer IP Law you can navigate the increasingly global practice of IP law with specialized, local and cross-border information and tools from every preferred location. Are you, as an IP professional, ready for the future?
Learn how Kluwer IP Law can support you.
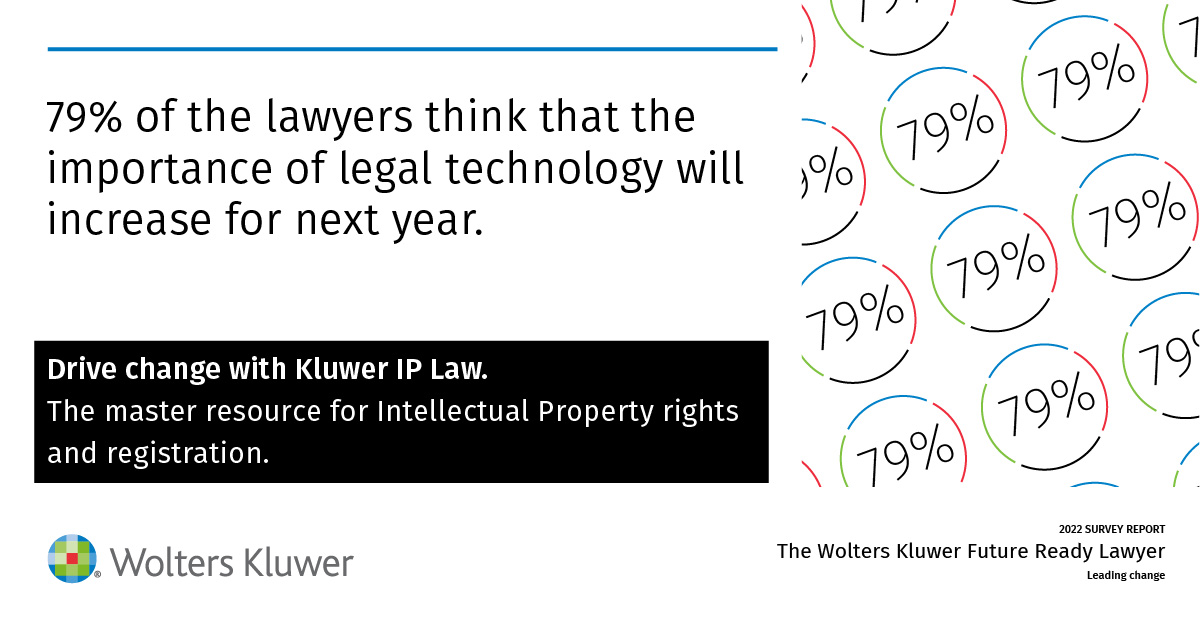

Brillant and well-documented article!